Catherine Dong
Major tech companies have actively reoriented themselves around AI and machine learning: Google is now “AI-first,” Uber has ML running through its veins and internal AI research labs keep popping up.
They’re pouring resources and attention into convincing the world that the machine intelligence revolution is arriving now. They tout deep learning, in particular, as the breakthrough driving this transformation and powering new self-driving cars, virtual assistants and more.
Despite this hype around the state of the art, the state of the practice is less futuristic.
Software engineers and data scientists working with machine learning still use many of the same algorithms and engineering tools they did years ago.
That is, traditional machine learning models — not deep neural networks — are powering most AI applications. Engineers still use traditional software engineering tools for machine learning engineering, and they don’t work: The pipelines that take data to model to result end up built out of scattered, incompatible pieces. There is change coming, as big tech companies smooth out this process by building new machine learning-specific platforms with end-to-end functionality.
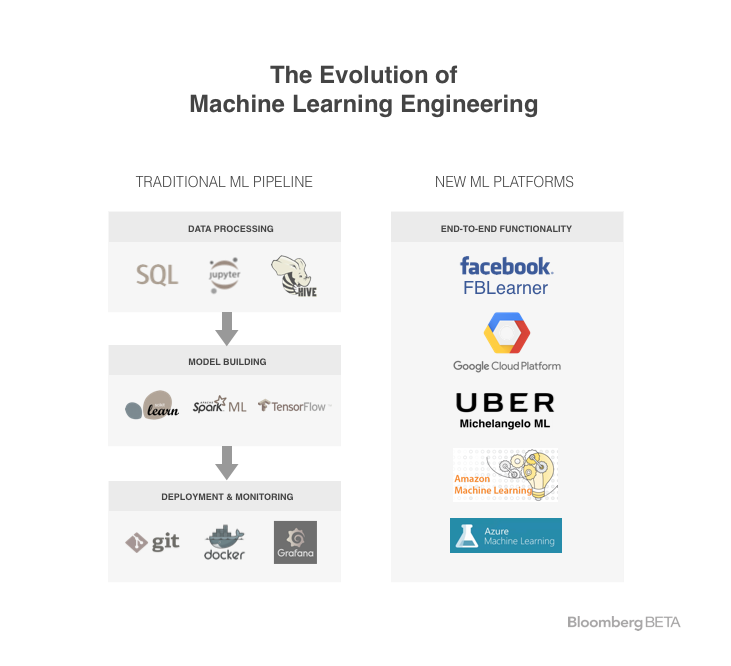
What goes into a machine learning sandwich
Machine learning engineering happens in three stages — data processing, model building and deployment and monitoring. In the middle we have the meat of the pipeline, the model, which is the machine learning algorithm that learns to predict given input data.
That model is where “deep learning” would live. Deep learning is a subcategory of machine learning algorithms that use multi-layered neural networks to learn complex relationships between inputs and outputs. The more layers in the neural network, the more complexity it can capture.
Traditional statistical machine learning algorithms (i.e. ones that do not use deep neural nets) have a more limited capacity to capture information about training data. But these more basic machine learning algorithms work well enough for many applications, making the additional complexity of deep learning models often superfluous. So we still see software engineers using these traditional models extensively in machine learning engineering — even in the midst of this deep learning craze.
But the bread of the sandwich process that holds everything together is what happens before and after training the machine learning model.
The first stage involves cleaning and formatting vast amounts of data to be fed into the model. The last stage involves careful deployment and monitoring of the model. We found that most of the engineering time in AI is not actually spent on building machine learning models — it’s spent preparing and monitoring those models.
The meat of machine learning — and avoiding exotic flavors
Despite the focus on deep learning at the big tech company AI research labs, most applications of machine learning at these same companies do not rely on neural networks and instead use traditional machine learning models. The most common models include linear/logistic regression, random forests and boosted decision trees. These are the models behind, among other services tech companies use, friend suggestions, ad targeting, user interest prediction, supply/demand simulation and search result ranking.
And some of the tools engineers use to train these models are similarly well-worn. One of the most commonly used machine learning libraries is scikit-learn, which was released a decade ago (although Google’s TensorFlow is on the rise).
There are good reasons to use simpler models over deep learning. Deep neural networks are hard to train. They require more time and computational power (they usually require different hardware, specifically GPUs). Getting deep learning to work is hard — it still requires extensive manual fiddling, involving a combination of intuition and trial and error.
With traditional machine learning models, the time engineers spend on model training and tuning is relatively short — usually just a few hours. Ultimately, if the accuracy improvements that deep learning can achieve are modest, the need for scalability and development speed outweighs their value.
Attempting to stick it all together — tools from data to deployment
So when it comes to training a machine learning model, traditional methods work well. But the same does not apply to the infrastructure that holds together the machine learning pipeline. Using the same old software engineering tools for machine learning engineering creates greater potential for errors.
The first stage in the machine learning pipeline — data collection and processing — illustrates this. While big companies certainly have big data, data scientists or engineers must clean the data to make it useful — verify and consolidate duplicates from different sources, normalize metrics, design and prove features.
At most companies, engineers do this using a combination SQL or Hive queries and Python scripts to aggregate and format up to several million data points from one or more data sources. This often takes several days of frustrating manual labor. Some of this is likely repetitive work, because the process at many companies is decentralized — data scientists or engineers often manipulate data with local scripts or Jupyter Notebooks.
Furthermore, the large scale of big tech companies compounds errors, making careful deployment and monitoring of models in production imperative. As one engineer described it, “At large companies, machine learning is 80 percent infrastructure.”
However, traditional unit tests — the backbone of traditional software testing — don’t really work with machine learning models, because the correct output of machine learning models isn’t known beforehand. After all, the purpose of machine learning is for the model to learn to make predictions from data without the need for an engineer to specifically code any rules. So instead of unit tests, engineers take a less structured approach: They manually monitor dashboards and program alerts for new models.
And shifts in real-world data may make trained models less accurate, so engineers re-train production models on fresh data on a daily to monthly basis, depending on the application. But a lack of machine learning-specific support in the existing engineering infrastructure can create a disconnect between models in development and models in production — normal code is updated much less frequently.
Many engineers still rely on rudimentary methods of deploying models to production, like saving a serialized version of the trained model or model weights to a file. Engineers sometimes need to rebuild model prototypes and parts of the data pipeline in a different language or framework, so they work on production infrastructure. Any incompatibility from any stage of the machine learning development process — from data processing to training to deployment to production infrastructure — can introduce error.
Making it presentable — the road forward
To address these issues, a few big companies, with the resources to build custom tooling, have invested time and engineering effort into creating their own machine learning-specific tools. Their goal is to have a seamless, end-to-end machine learning platform that is fully compatible with the company’s engineering infrastructure.
Facebook’s FBLearner Flow and Uber’s Michelangelo are internal machine learning platforms that do just that. They allow engineers to construct training and validation data sets with an intuitive user interface, decreasing time spent on this stage from days to hours. Then, engineers can train models with (more or less) the click of a button. Finally, they can monitor and directly update production models with ease.
Services like Azure Machine Learning and Amazon Machine Learning are publicly available alternatives that provide similar end-to-end platform functionality but only integrate with other Amazon or Microsoft services for the data storage and deployment components of the pipeline.
Despite all the emphasis big tech companies have placed on enhancing their products with machine learning, at most companies there are still major challenges and inefficiencies in the process. They still use traditional machine learning models instead of more-advanced deep learning, and still depend on a traditional infrastructure of tools poorly suited to machine learning.
Fortunately, with the current focus on AI at these companies, they are investing in specialized tools to make machine learning work better. With these internal tools, or potentially with third-party machine learning platforms that are able to integrate tightly into their existing infrastructures, organizations can realize the potential of AI.
A special thank you to Irving Hsu, David Eng, Gideon Mann and the Bloomberg Beta team for their insights.
Comment