Machine learning can provide companies with a competitive advantage by using the data they’re collecting — for example, purchasing patterns — to generate predictions that power revenue-generating products (e.g. e-commerce recommendations). But it’s difficult for any one employee to keep up with — much less manage — the massive volumes of data being created. That poses a problem, given AI systems tend to deliver superior predictions when they’re provided up-to-the-minute data. Systems that aren’t regularly retrained on new data run the risk of becoming “stale” and less accurate over time.
Fortunately, an emerging set of practices dubbed “MLOps” promises to simplify the process of feeding data to systems by abstracting away the complexities. One of its proponents is Mike Del Balso, the CEO of Tecton. Del Balso co-founded Tecton while at Uber when the company was struggling to build and deploy new machine learning models.
“Models that are provided with highly refined real-time features can deliver much more accurate predictions. But building data pipelines to generate these features is hard, requires significant data engineering manpower, and can add weeks or months to project delivery times,” Del Balso told TechCrunch in an email interview.
Del Balso — who previously led Search ads machine learning teams at Google — co-launched Tecton in 2019 with Jeremy Hermann and Kevin Stumpf, two former Uber colleagues. While at Uber, the trio had created Michelangelo, an AI platform that Uber used internally to generate marketplace forecasts, calculate ETAs and automate fraud detection, among other use cases.
The success of Michelangelo inspired Del Balso, Hermann and Stumpf to create a commercial version of the technology, which became Tecton. Investors followed suit. Case in point, Tecton today announced that it raised $100 million in a Series C round that brings the company’s total raised to $160 million. The tranche was led by Kleiner Perkins, with participation from Databricks, Snowflake, Andreessen Horowitz, Sequoia Capital, Bain Capital Ventures and Tiger Global. Del Balso says it’ll be used to scale Tecton’s engineering and go-to-market teams.
“We expect the software we use today to be highly personalized and intelligent,” Kleiner Perkins partner Bucky Moore said in a statement provided to TechCrunch. “While machine learning makes this possible, it remains far from reality as the enabling infrastructure is prohibitively difficult to build for all but the most advanced companies. Tecton makes this infrastructure accessible to any team, enabling them to build machine learning apps faster.”
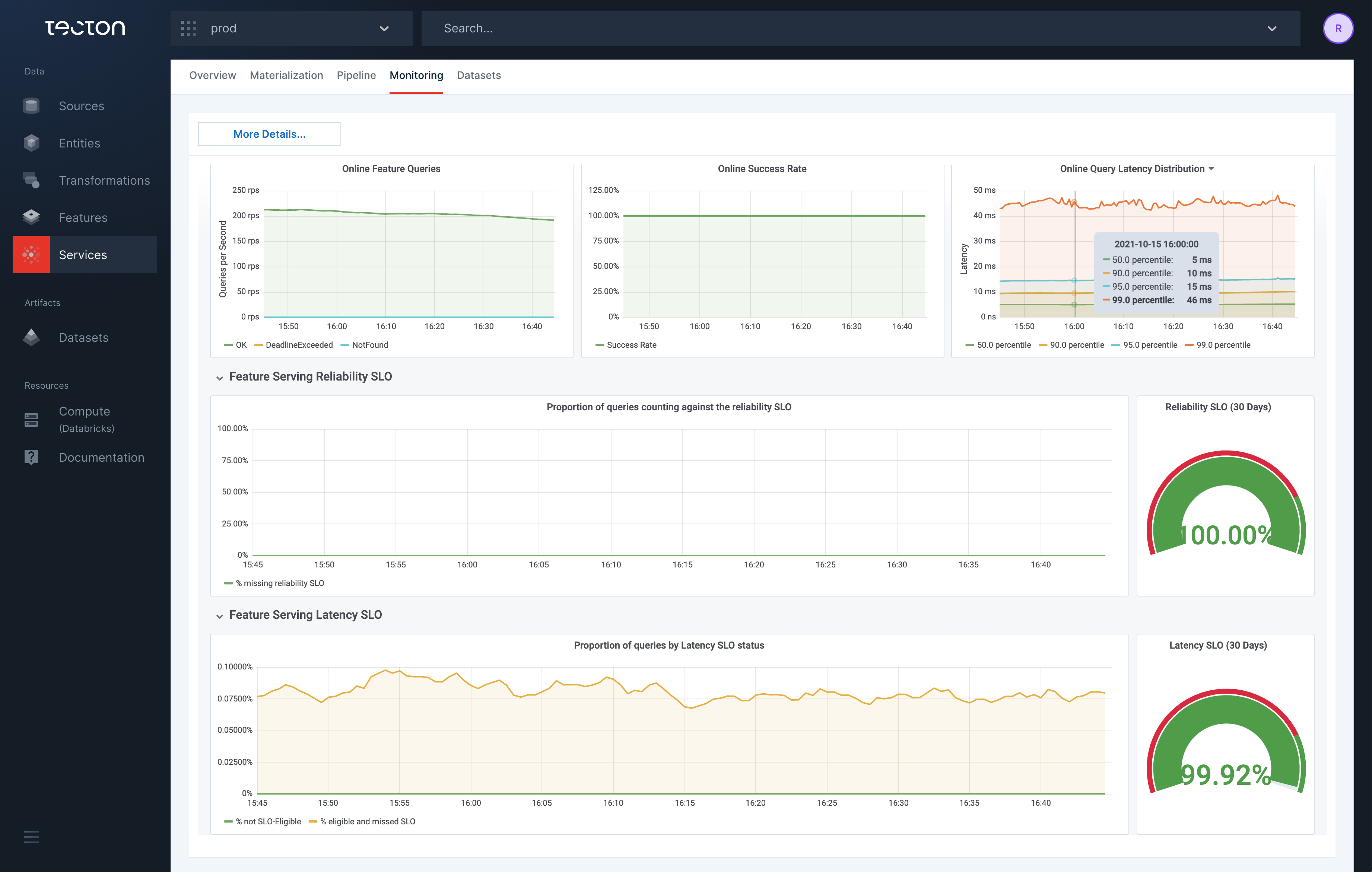
Tecton’s monitoring dashboard. Image Credits: Tecton
At a high level, Tecton automates the process of building features using real-time data sources. “Features,” in machine learning, are individual independent variables that act like an input in an AI system. Systems use features to make their predictions.
“[Automation,] allows companies to deploy real-time machine learning models much faster with less data engineering effort,” Del Balso said. “It also enables companies to generate more accurate predictions. This can in turn directly translate to the bottom line, for example by increasing fraud detection rates or providing better product recommendations.”
In addition to orchestrating data pipelines, Tecton can store feature values across AI system training and deployment environments. The platform can also monitor data pipelines, calculating the latency and processing costs, and retrieve historical features to train systems in production.
Tecton also hosts an open source feature store platform, Feast, that doesn’t requiring dedicated infrastructure. Feast instead reuses existing cloud or on-premises hardware, spinning up new resources when needed.
“Typical use cases for Tecton are machine learning applications that benefit from real-time inference. Some examples include fraud detection, recommender systems, search, underwriting, personalization, and real-time pricing,” Del Balso said. “Many of these machine learning models perform much better when making predictions in real-time, using real-time data. For example, fraud detection models are significantly more accurate when using data on a user’s behavior from just a few seconds prior, such as number, size, and geographical location of transactions.”
According to Cognilytica, the global market for MLOps platforms will be worth $4 billion by 2025 — up from $350 million in 2019. Tecton isn’t the only startup chasing after it. Rivals include Comet, Weights & Biases, Iterative, InfuseAI, Arrikto and Continual to name a few. On the feature store front, Tecton competes with Rasgo and Molecula, as well as more established brands like Google and AWS.
Del Balso points to a few points in Tecton’s favor, like strategic partnerships and integrations with Databricks, Snowflake and Redis. Tecton has hundreds of active users — no word on customers, other than the fact that the base quintupled over the past year — and Del Balso said that gross margins (net sales minus the cost of goods sold) are above 80%. Annual recurring revenue apparently tripled from 2021 to 2022, but Del Balso declined to provide firm numbers.
“We are still in the early innings of MLOps. This is a difficult transition for enterprises. Their teams of data scientists have to behave more like data engineers and start building production-quality code. They need a whole set of new tools to support this transition, and they need to integrate these tools into coherent machine learning platforms. The ecosystem of MLOps tools is still highly fragmented, making it more difficult for enterprises to build these machine learning platforms,” Del Balso said. “The pandemic accelerated the transition to digital experiences, and with that the importance of deploying operational ML to power these experiences. We believe that the pandemic was an accelerator for the adoption of new MLOps tools, including feature stores and feature platforms.”
San Francisco-based Tecton currently has 80 employees. The company plans to hire about 20 over the next six months.