By Seva Vayner, Director of Edge Cloud at G-Core Labs
More and more businesses are looking to implement artificial intelligence and machine learning into their processes and solutions. Machine learning models can impact businesses across all verticals, helping them unlock new insights, reduce operational costs, improve their customer experience and so much more. But AI integration can be complex and organisations hoping to utilise the technology are faced with many hurdles to overcome before they can see the benefits. The first obstacle is getting the right IT infrastructure in place, AI requires processing power, storage and more to support it, and for smaller enterprises having the tech stack to support AI can seem like an insurmountable obstacle.
That’s why G-Core Labs, an international provider of cloud and edge solutions, has launched a new AI-infrastructure-as-a-service solution within its cloud platform. The AI Cloud is designed to support businesses of all sizes across every stage of their AI adoption journey. The cloud infrastructure includes powerful bare-metal servers, Graphcore Intelligence Processing Units (IPUs) specifically designed for AI, and lots of integrated tools and resources to make developing AI applications easier for developers and data scientists across finance, fin-tech, e-commerce and game development.
Let’s take a closer look at the reasons why this AI-infrastructure-as-a-service is the future of AI & ML inference & training.
Preparing and storing data
Artificial intelligence, especially machine learning algorithms, requires a lot of data. One of the first hurdles for a team developing AI applications is simply collecting, preparing and storing these data sets in a cost-effective way. The companies that start off their data collection with paper ledgers and end up with endless .csv files will have a far harder time with data preparation than a team with a smaller but ML-friendly dataset.
Some organisations have been hoarding data records for decades which, if stored in on-site servers, require a lot of resources to build into an AI application or migrate to the cloud. That’s not to mention the potential compliance challenges. This is one of the advantages of the G-Core Labs AI cloud infrastructure. Teams can start preparing data correctly for machine learning right away, with no manual effort whatsoever. By simply storing data in cloud storage, the AI project will automatically have access to the data required for modelling. On top of this, G-Core infrastructure is built with all steps of the process in mind, from storing to building, to labelling and training the AI models.
Build notebooks and accelerate the ML lifecycle
Beyond tools and infrastructure to simply store data, G-Core Labs AI Cloud comes with tools to help create and train models, built into the platform. The AI Cloud allows you to easily create and run Jupiter notebooks within the cloud, allowing teams to build out AI solutions easily – you could even easily automate the integration of ML code changes from multiple developers into a single project.
On top of this, the platform comes with ready-to-use tools, templates and models for developing ML code and training ML models. Our marketplace within the platform is a one-stop shop with all the tools to suit the needs and requirements of your current AI project. These tools can support data science teams and save resources on unnecessary manual coding from square one – just choose a pre-built model and customise it from there. This combined with ready-to-go infrastructure can give your team a leg-up and accelerate the rollout or testing of any AI application.
Build ML models backed up by powerful Graphcore hardware
The demanding tasks that enterprises and innovators look to use ML for, such as next generation, higher accuracy computer vision, natural language processing and graph ML, require a powerful platform that gives faster time to results. CPUs and GPUs aren’t always the best choice for such tasks leaving competitive advantage on the table. This is why G-Core Labs uses ground-breaking Intelligence Processing Units (IPUs) systems from Graphcore, designed from the ground up for AI.
Let’s look at some benchmarks:
- The Graphcore IPU was tested not just with MLPerf applications but ran through a range of Natural Language Processing, higher accuracy Computer Vision and Graph ML models, including EfficientNet, vision transformer (ViT), Dynamic Temporal Graph Networks and Generative Pre-trained Transformers (GPT). Benchmark results highlight excellent scaling which is key for large-scale ML deployments and validates the hardware and software design.
- Absolute performance was also impressive, training EfficientNet-B4 on a Bow Pod16 in less than 14 hours, compared to the latest GPU technology at 70.5 hours.
- Dynamic Temporal Graph Networks, suitable for applications in social networks, financial transactions and recommender systems see a 10 x higher performance compared to GPU
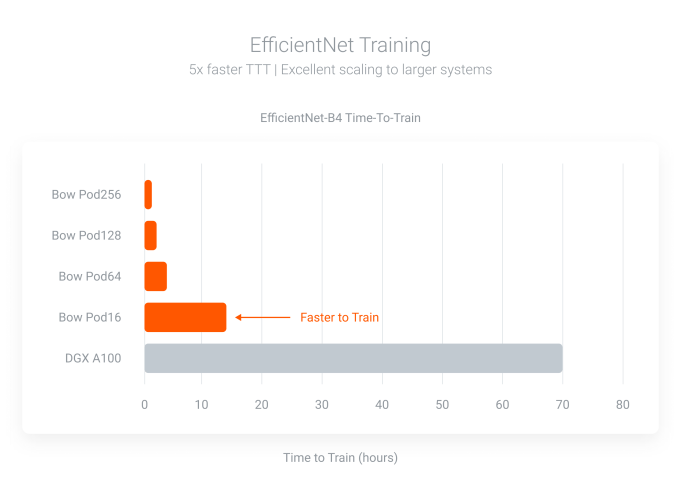
Image Credits: G-Core Labs
This hardware is so powerful that it is being used for accelerating fusion energy research at Hartree Centre, a world-leading supercomputing facility. The research centre uses Graphcore IPUs to deliver clean and commercially viable fusion energy, as part of its mission to transform UK industry through high-performance computing (HPC), data analytics, and AI.
Graphcore IPUs have been proven to be far more powerful processors for AI/ML tasks, meaning AI applications running via this hardware will perform tasks faster and more cost efficiently than alternative architectures. G-Core AI Cloud gives you easy access to these IPUs.
Inference close to your users
Once you have built your ML model, it’s time to consider inference – where the model will actually get to work processing data and doing what it has been trained for. For real-time applications such as facial recognition or the detection of defective products in production, the result needs to be generated and returned as quickly as possible – this is where AI inference at the edge comes in.
While you can carry out inference in the cloud, it’s more optimal to base it from a device local to the data being analysed, rather than transferring data back and forth. This can significantly reduce the time for a result to be generated – therefore for real-time use cases such as facial recognition, inference at the edge is non-negotiable.
Again, this is somewhere that the G-Core Labs Cloud infrastructure can help enterprises. G-Core has local cloud points of presence all over the world, meaning you can ensure inference takes place closer to your data source. Using local points of presence also makes it far easier to meet local data compliance and legislative requirements.
Conclusion
As digital businesses move closer to industry 4.0, technologies like AI/ML and cloud computing will be crucial in helping enterprises to reduce costs, utilise data and unlock more value than ever before. G-Core Labs Cloud allows businesses to cover both of these bases with a leading global AI infrastructure.
Using this, developers and data teams can accelerate their projects by ensuring all required infrastructure is secured and of the highest quality and will be able to take advantage of tools to support them in every step of the process. G-Core Labs AI cloud democratises AL & ML technologies and gives even the smallest start-ups access to the IT resources of a tech giant. You can explore more here.