By Marco Casalaina (@amrcn_werewolf), GM of Einstein, @Salesforce
Automation is all the rage lately. Thirty five years ago, my father, Joseph Casalaina, developed some of the first automation systems for the insurance company MetLife, in the form of “expert systems.” Expert systems allowed programmers to create business rules that systems would follow, reducing the need for human intervention, while preventing errors. Large companies had entire divisions of programmers devoted to creating and maintaining these rules.
Today, we find ourselves in the brave new world of artificial intelligence (AI) and automation tools that anyone can use, and they’re becoming more powerful and accessible than ever. And, it’s no surprise companies are racing to adopt them as quickly as possible. It’s no longer a matter of if AI and automation will change how businesses operate, but how and when. For example, institutions such as Academy Bank and nCino used low-code tools and automated workflows to stand up an application process in a matter of days.
And yet I see many companies of all sizes that, after considerable investments, fail to make the most of the technology. Maybe it’s because of movies like Her, which make AI look all-powerful, but too many assume the technology will immediately solve all their problems. Unfortunately, technology isn’t there yet – maybe (dare I say, hopefully?) it never will be.
The recent Trends in Workflow Automation Report shows that 95% of IT and engineering leaders say their organizations are prioritizing workflow automation – and with modern automation tools, that also means AI.
In the rush to transform our businesses, though, we often forget to stop and figure out just what we hope to achieve, and how these tools can help get us there.
Here are four of the most important rules to follow to get the most from your workflow automation projects:
1. Ask what you really want the technology to deliver
It’s important to start by clearly defining the tangible outcomes we expect. As my colleague Sarah Aerni explained, it’s not just about having a fancy AI model, it’s about what it does for you and what value it can bring to your business. With tangible outcomes identified, you can choose the right tools for the job.
Then, when it comes to choosing solutions, focus on AI that seamlessly integrates with existing systems and processes. AI is increasingly built into common software tools, and it’s often easiest to work with what you already have in place. Plus, your users will already be familiar with these systems, which engenders trust.
With outcomes identified and tools in place, the next step is to look for friction points – areas where repetitive, manual tasks lower the return on investment (ROI). It’s important to start small and in an area which will demonstrate a quick win. Start with focusing on areas where you could help remove grunt work from employees’ plates. I’ve seen too many businesses think they need to tackle every process at once, and that never works. The old adage “don’t bite off more than you can chew” resonates as much when it comes to technology as it does to life.
2. Find out where people and processes intersect
As Kai Nunez mentioned in her TechCrunch article about designing automation technology that partners with humans, it’s critical to seek out diverse perspectives during your process planning. However, don’t stop there. When implementing your workflows, it’s just as important to make sure everyone involved is onboard and feels invested in its success. This approach will also play in your favor when it comes to getting the most success out of any change management initiative.
A key step here is deciding when to bring in the “human in the loop.” For example, if you’re using a computer vision system to process a customer payment, and it has low confidence in whether it read the payment correctly, the process generally should not just block the payment from going through. Instead it should transfer it to a human. Similarly, chatbots can automatically answer many questions but are often configured to route questions to a human agent if it is unable to answer a question. Companies that want to get the most out of their AI and automation investments should plan in advance for the “human in the loop.” It’s crucial to make sure the right teams are in place to not only set up the technology, but also facilitate continuous learning and measure its impact.
3. Perform a pilot before rolling it out into production
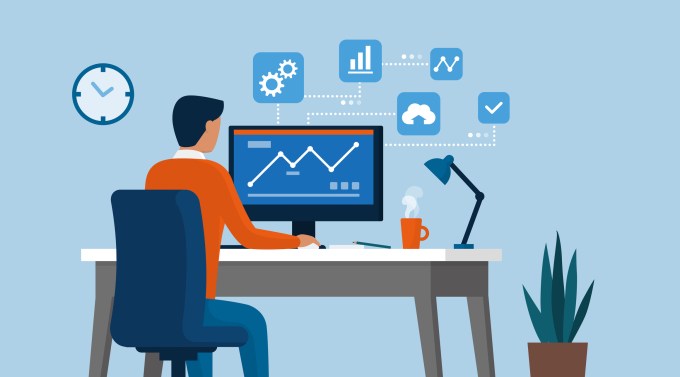
Image Credits: Getty Images
Technically, you could stay in planning mode as long as you want. However, your competitors are likely working on their own AI and automation strategies, so time is of the essence. The best way to learn is by trying, measuring, assessing the results and trying again.
Successful AI rollouts often involve significant pilot periods. During these pilot periods, a best practice is to select a subset of users who will use the new AI-guided process. The purpose of this pilot is to understand whether the AI model itself is effective, but also to learn whether your users understand, trust and can work with the AI.
A pilot period also gives you the ability to do a direct comparison between an AI-guided process and a manual process. Is it working? How much time is it saving your users? How much money is it saving (or making) your business? By comparing the pilot users to the non-pilot set, you can get an early idea about the benefits.
4. Measure the impact and ROI
Let’s say everything looks good during your pilot period, and you’ve decided to roll out your new AI-guided process more broadly. It’s important to continue measuring its impact.
Investments in AI and automation can be costly. While these investments more than pay off in reducing operational costs, many companies don’t actually track the ROI. They have no idea whether the cost of purchasing software, paying for data storage and computing and hiring data scientists actually has an impact on the bottom line. Failing to measure ROI can lead to missed opportunities to prove the value from pilot implementations that would have otherwise driven greater value for your organization.
The first step to measuring ROI goes back to setting clear goals for your AI and automation projects.
Then, for each goal, identify the key performance indicators (KPIs) that align with those business goals. Be sure to measure the baseline of these KPIs: what did they look like before the AI was implemented? Once you’ve broadly deployed the AI, regularly measure the impact against your benchmarks to track progress, or define areas for improvement.
Knowing how well your AI and automation tools are working is critical to sustaining projects for the long haul, justifying their ongoing costs and maximizing their value. The days of “set it and forget it” solutions have not yet arrived, which is why continuous measurement is so important.
Finally, keep in mind what matters.
AI is everywhere today, but it does not act alone. Nearly every AI system in operation today is paired with an automation system that turns AI guidance into actions that humans can understand. In my father’s day, “automation” meant that programmers had to manually program every rule, every keyword and every action. But today, automation is paired with AI, which can learn to understand language, predict behavior, or make recommendations – making automation more about “learning” and less about “programming.”
Still, for all the things AI and automation can do, it is up to us — the humans — to make the important decisions.