By Florian Douetteau, Dataiku
For all the potential artificial intelligence holds for everyday business operations, the vast majority of today’s enterprises still struggle to realize value from their investments in the technology. While the giants leverage their near-infinite resources to build powerful algorithms into their platforms, most companies are left to their own devices, implementing either a complex web of various third-party technologies, or building sub-optimal models of their own, often based on incomplete or inaccurate data.
In my eight years at Dataiku, I’ve seen thousands of promising companies waylaid by impractical or inefficient attempts to build AI systems that provide real value. The reality is, AI can help businesses in any industry, but companies that fail to plan for common pitfalls find themselves in a tough position, with runaway costs, potential biases or false returns. While there are myriad considerations to take into account when implementing AI in the enterprise, here are the five key reasons that your organization may be failing at AI – and how to right the ship before it’s too late.
Choose your use case wisely (and don’t reinvent the wheel)
Companies often fall into the trap of assuming they can drop an algorithm into any process and see immediate results. Executives and teams that are considering an investment in AI — whether building projects internally or outsourcing to external vendors — should consider the results they want to see, and how they expect to achieve them with AI, before kicking off any project. Does your company need to build a better customer recommendation engine, or improve a fraud detection system? These are very different questions with very different answers – having a clear vision for what your organization is trying to achieve will help the entire enterprise align on costs, deadlines and methodology, and plays an important role in avoiding future pitfalls.
The best enterprises don’t just go in with a plan, they leverage existing algorithms and models to supercharge progress and drive down costs. Anyone who has worked with AI knows that training and developing models comes with a price tag — one estimate pegged the cost of training GPT-3 at nearly $5 million, an investment that most organizations couldn’t dream of making, and one that ignores the trial and error that goes into the development of any good model.
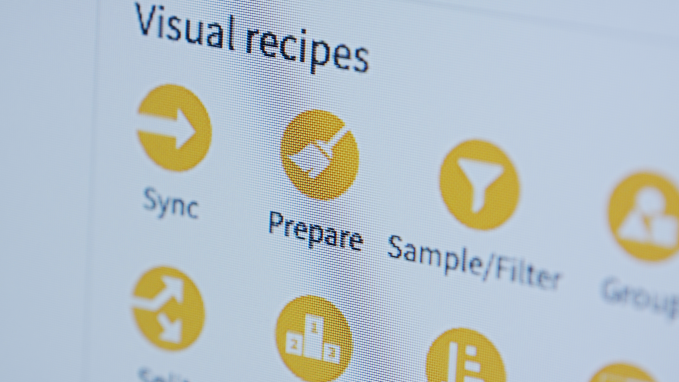
Image Credits: Dataiku (opens in a new window)
The economics of AI development mean that enterprises can and should reuse existing assets already in production, such as cleaned and prepped datasets — for most projects, it’s not necessary to start from absolute zero. Think of it like any open source project: why write software from scratch when there is existing code that will do the thing you need it to?
Always question your data — but don’t let your questions hold you back
“An algorithm is only as good as the data that feeds it” is a common refrain for good reason, yet so many organizations kick off an AI project without a clear strategy for ensuring the quality of their data. Data quality isn’t a one-time consideration — extensive work must be done before, during and after model development to ensure that datasets in use are comprehensive, up to date and accurate, and that as much bias as possible is removed or accounted for. Of course, waiting for “perfect” datasets means you’ll likely never kick off a project — there’s simply too many variables that can change and outliers that can be introduced. The recent release of Dataiku 9 included tools like Smart Pattern Builder and Fuzzy Joins, which give business analysts the ability to work with more complex (or incomplete) datasets without having to write code or manually clean and prepare data.

Image Credits: Dataiku (opens in a new window)
This comes down to a successful data governance strategy, and selecting tools that enable governance easily. Executive leadership is also critical — without top-down buy-in, governance slips, and the downsides can be enormous: in less than four years, Europe’s General Data Protection Regulation (GDPR) has resulted in more than $600 million in fines, all related to issues of data governance and privacy. Dataiku’s platform provides immense help here, with extensive built-in governance that complies with any and all global regulations – especially critical for customers that work in regions with vastly different data privacy laws.
Transparency and clear communication (as well as a willingness to adjust) are key, as is creating comprehensive, sustainable governance frameworks that all areas of a company can follow. In recent years, the idea of AI governance has become increasingly commonplace, and in 2019, Singapore became the first country to adopt an overarching framework for AI governance — I expect to see more companies (and countries) follow in their footsteps and prioritize an ongoing governance strategy, and enterprises will continue to look for ways to make governance a baked-in element of any AI initiative.
Don’t forget who you’re building for
A content recommendation engine or churn prediction model at the heart of a company’s operations cannot just be APIs exposed from a data scientist’s notebook — they require full operationalization after their initial design. When AI projects aren’t effectively incorporated into business processes, and held to KPIs that align with the business unit they are servicing, ROI is hard to come by. Our most recent platform update, Dataiku 9.0, introduced a number of new features to help AI projects further integrate with business goals, particularly with ML Assertions — this allows true subject matter experts to embed known sanity-checks into the model to catch instances where typical model metrics might lead to a sense of false security.
Operationalization takes planning and execution, as well as a recognition across the enterprise that successful AI projects take time to build and deploy. Lines of business and subject matter experts must be involved in the development and operationalization process — operationalization happening in a vacuum without any input from business teams is doomed to fail, delivering projects that don’t address real needs, or do so superficially.
Maintenance never stops
Many organizations, especially those new to AI implementation, view the technology as a “point and click” solution — a user can simply “turn on” their AI model and watch it work perfectly, in perpetuity. The reality is just the opposite: strong AI models require constant maintenance and monitoring, as well as frequent audits of inputs and outputs, in order to ensure that the models are responsible, transparent and accurate. The concept of model drift (or model decay) sounds quite technical, but it’s rather simple — as variables change over time, an AI model’s predictive power will weaken unless the model is tweaked and updated to reflect the current environment from which it is drawing data.
The onset of Covid-19 has made model drift somewhat mainstream (it’s easy to understand why suddenly closing 300 Apple stores would leave an algorithm unable to predict in-person sales), but most organizations still lack real processes and procedures for combatting it. It is here that MLOps becomes critical — collaboration across all relevant teams is necessary to ensure that variable changes are accounted for. There are also a number of tools on the market that help engineers manage model drift with less active oversight — Dataiku’s Model Drift Monitoring feature provides views to analyze the potential drift of machine learning models, and is free for all enterprise users.

Image Credits: Dataiku (opens in a new window)
Don’t build in a silo
“Democratization of AI” has reached buzzword status, but that shouldn’t stop organizations from trying to get more personas involved in AI development. Too many companies view data scientists as the only people that should ever build or interact with AI models, but this mindset is limiting. Data scientists are brilliant people, but they can’t be expected to be experts in every industry they serve. Subject matter experts should be brought into the development process, and business analysts and executives alike should play a role in defining KPIs and operationalizing models in production. Down the line, tools like Dataiku’s what-if analysis can play an important role in helping decision-makers interact with (and request changes to) AI models, enabling the C-suite to view the potential impact of real-time changes to input data.

Image Credits: Dataiku (opens in a new window)
AI was once seen as an easy-to-use, plug-and-play technology, but it’s become a fragmented web of third party tools that require extensive human intervention to provide any value. Companies have swung between seeking all-in-one solutions, or deploying multiple best-of-breed tools, but successful AI implementations are just as reliant on culture as they are on technology. Focusing on specific projects that provide tangible benefits to the bottom line, encouraging collaboration across teams and regions, and keeping transparency and responsibility in mind will lead companies to success.
Is your organization struggling to implement AI in the enterprise, or to empower new roles to work with data in meaningful ways? Dataiku can help. To learn more about Dataiku’s platform or start a free trial, visit www.dataiku.com.