It’s a wonder what generative AI, particularly text-to-image AI models like Midjourney and OpenAI’s DALL-E 3, can do. From photorealism to cubism, image-generating models can translate practically any description, short or detailed, into art that might well have emerged from an artist’s easel.
The trouble is, many of these models — if not most — were trained on artwork without artists’ knowledge or permission. And while some vendors have begun compensating artists or offering ways to “opt out” of model training, many haven’t.
In lieu of guidance from the courts and Congress, entrepreneurs and activists are releasing tools designed to enable artists to modify their artwork so that it can’t be used in training GenAI models. One such tool, Nightshade — released this week — makes subtle changes to the pixels of an image to trick models into thinking the image depicts something different from what it actually does. Another, Kin.art, uses image segmentation (i.e., concealing parts of artwork) and tag randomization (swapping an art piece’s image metatags) to interfere with the model training process.
Launched today, Kin.art’s tool was co-developed by Flor Ronsmans De Vry, who co-founded Kin.art, an art commissions management platform, alongside Mai Akiyoshi and Ben Yu a few months ago.
As Ronsmans De Vry explained in an interview, art-generating models are trained on datasets of labeled images to learn the associations between written concepts and images, like how the word “bird” can refer to not only bluebirds but also parakeets and bald eagles (in addition to more abstract notions). By “disrupting” either the image or the labels associated with a given piece of art, it becomes that much harder for vendors to use the artwork in model training, he says.
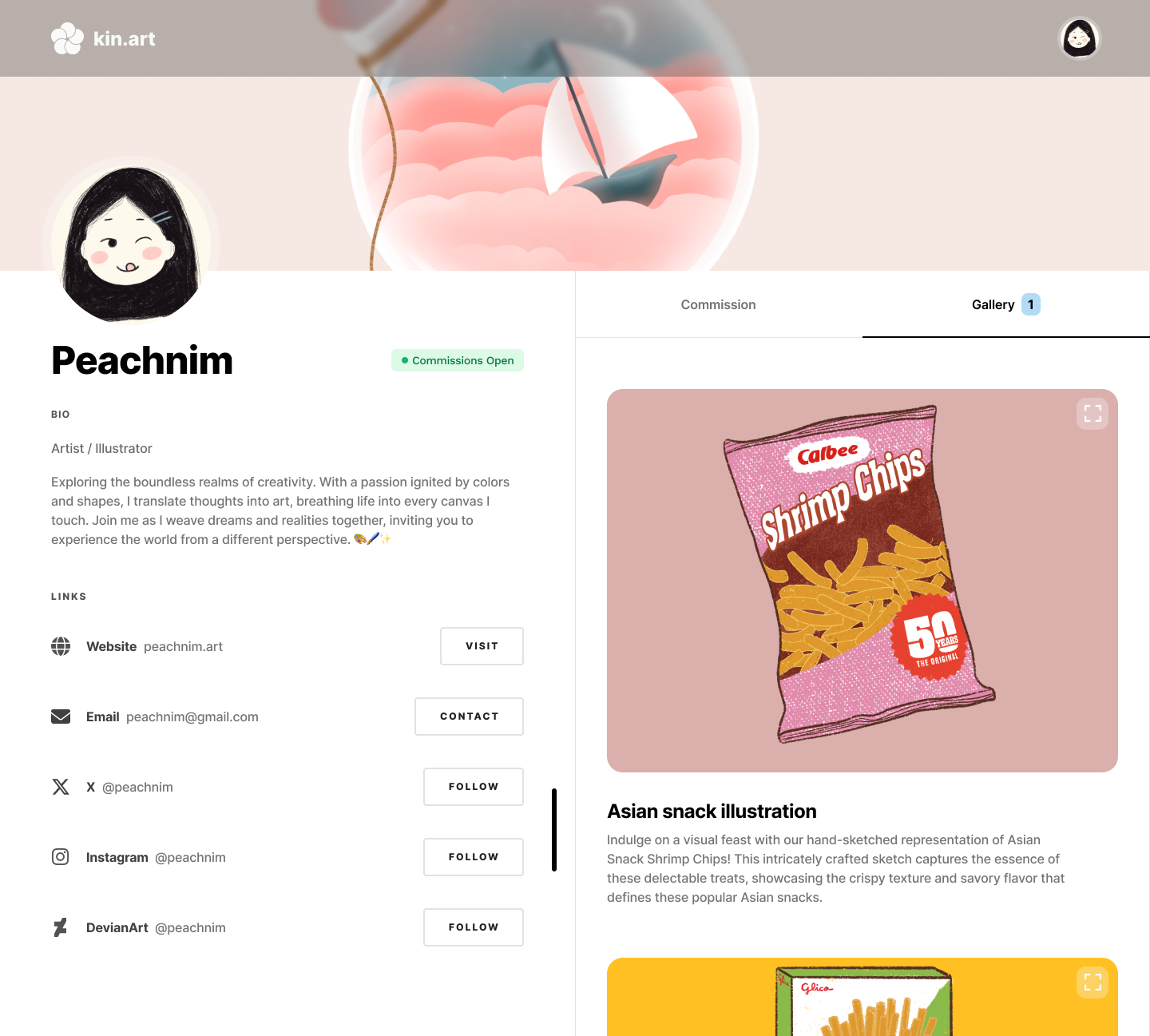
An artist profile on Kin.art. Image Credits: Kin.art
“Designing a landscape where traditional art and generative art can coexist has become one of the major challenges the art industry faces,” Ronsmans De Vry told TechCrunch via email. “We believe this starts from an ethical approach to AI training, where the rights of artists are respected.”
Ronsmans De Vry asserts that Kin.art’s training-defeating tool is superior in some ways to existing solutions because it doesn’t require cryptographically modifying images, which can be expensive. But, he adds, it can also be combined with those methods as additional protection.
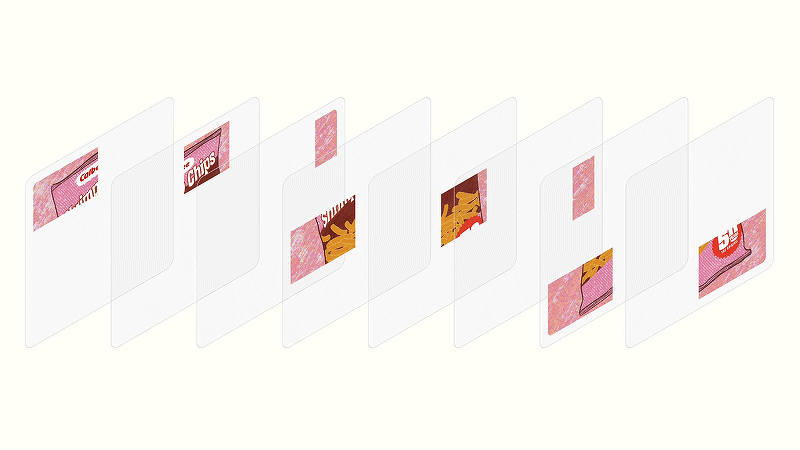
Kin.art’s model-defeating segmentation method. Image Credits: Kin.art
“Other tools out there to help protect against AI training try to mitigate the damage after your artwork has already been included in the dataset by poisoning,” Ronsmans De Vry said. “We prevent your artwork from being inserted in the first place.”
Now, Kin.art has a product to sell. While the tool is free, artists have to upload their artwork to Kin.art’s portfolio platform in order to use it. The idea at present, no doubt, is that the tool will funnel artists toward Kin.art’s range of fee-based art commission-finding and -facilitating services, its bread-and-butter business.
But Ronsmans De Vry is positioning the effort as mostly philanthropic, pledging that Kin.art will make the tool available for third parties in the future.
“After battle-testing our solution on our own platform, we plan to offer it as a service to allow any small website and big platform to easily protect their data from unlicensed use,” he said. “Owning and being able to defend your platform’s data in the age of AI is more important than ever . . . Some platforms are fortunate enough to be able to gate their data by blocking non-users from accessing it, but others need to provide public-facing services and don’t have this luxury. This is where solutions like ours come in.”