It’s hard to fathom that the work of Reverend Thomas Bayes is still coming back to drive cutting edge advancements in AI, but that’s exactly what’s happening. DARPA-backed Gamalon is the latest carrier of the Bayesian baton, launching today with a solution to help enterprises better manage their gnarly unstructured data.
The world of enterprise is full of unstructured data. This includes product codes, SKUs, and text from sources not formally cataloged in spreadsheets. Organization opens doors for businesses to extract new insights from existing resources and processes.
Gamalon is releasing two products today for AWS, Azure and Google Cloud customers to help them with this problem. The first, Structure, converts paragraphs into structured data. The second, Match, de-duplicates and links these data rows.
The underlying technology powering these solutions differs from many typical machine learning approaches in the way it approaches prior knowledge. One way to think about this sort of Bayesian framework is in the context of a medical diagnosis.
Let’s say someone asks a doctor what they make of their cough. The doctor contemplates and decides that the person could either have a cold or lung cancer. After all, people suffering from both typically exhibit a cough. The missing information however is that very few people walk around with lung cancer while many more have colds.
Bayesian frameworks let us take that extra dimension of information into account and update it as new data is created to build models of the world — an ideal way to think about drawing conclusions with data. An oversimplified deep learning model might just use the symptom data of thousands of hospital patients and try to extrapolate the given ailment. The reality is that the two approaches aren’t quite this opposed, but the metaphor gets the idea across.
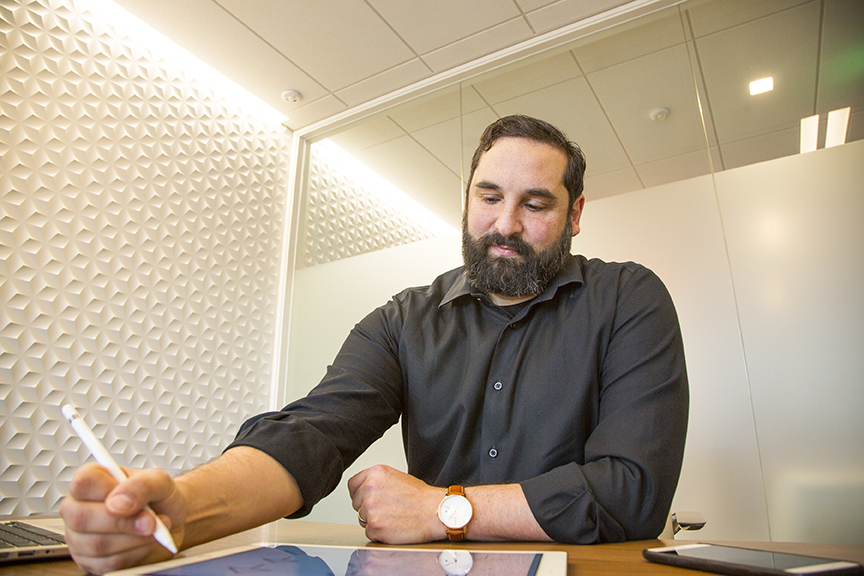
Founder Ben Vigoda
The result for Gamalon is a system that promises developers a clearer view of how models work. In contrast, deep learning models give us conclusions about data without much detail on what drives the analysis. Even still, both approaches have their ideal use cases — but historically the later has been given a lot more attention.
According to the company’s founder Ben Vigoda, Gamalon is writing neural networks as probabilistic programs, building sub-routines within neural nets to combine them with other trained models.
Collections of models can be easily combined to produce better results. This modularity enables a lot of problems to be solved with less data. The company is capitalizing on all of this by equipping computers to build models by themselves, a differentiating factor with respect to startups like Geometric Intelligence. Ideally humans and machines can work hand-in-hand. Fortunately for the humans, this ultimately places more value on domain knowledge and less value on pure mathematical prowess.
With the competitive advantage figured out, Gamalon next turned its head to commercialization. The startup trained a version of its framework on enterprise data and gave it a home in the cloud. Beta customers can use the system self-service and Gamalon will offer some professional services if necessary. Typical early customers have been e-commerce and manufacturing businesses that have massive amounts of unstructured data originating from a wide variety of places.
“Understanding unstructured data is a problem for 90 percent of enterprise companies,” asserted Aydin Senkut, a partner at Felicis Ventures. “A ton of audit money and human time is wasted looking for anomalies that a program could learn to find.”
To date, Felicis Ventures, Boston Seed Capital and Rivas Capital have lined up alongside angels like Adam D’Angelo, Andy Bechtolsheim, Steve Blank, Ivan Chong and Georges Harik to pour $4.45 million into the company. This comes on top of $7.7 million in government R&D contracts from DARPA for a total of $12.15 million in financing.